LangChain 记忆组件(学习笔记) 电脑版发表于:2023/9/16 18:28 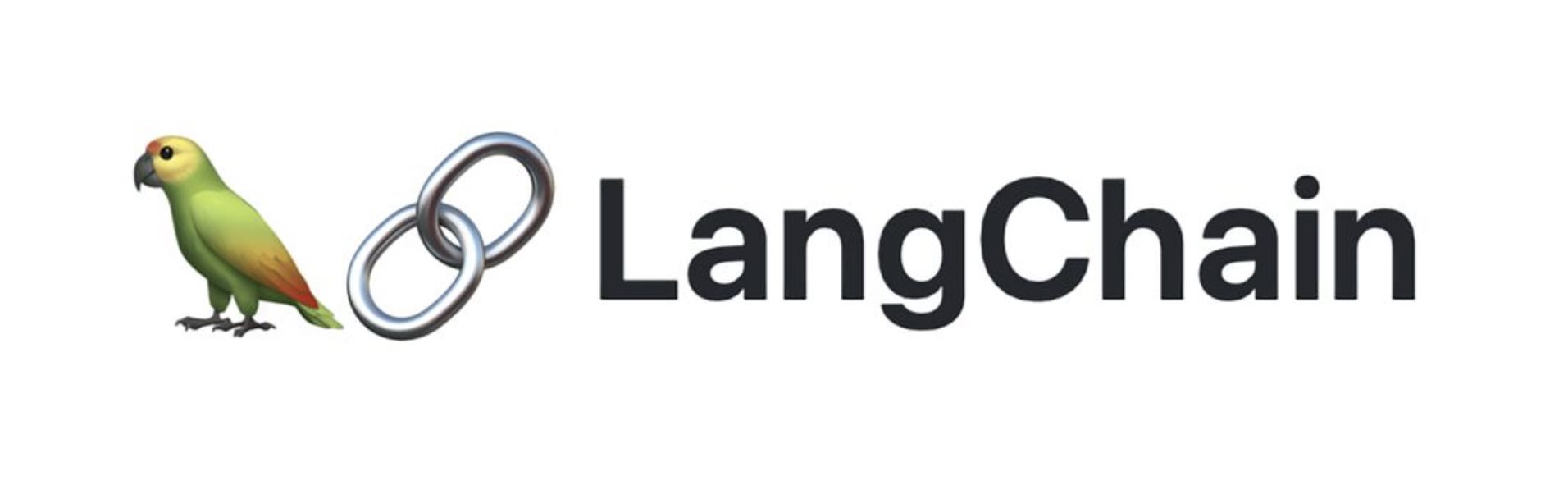 >#LangChain 记忆组件(学习笔记) [TOC] tn2>单一的LLM对于简单的应用场景已经足够,但是更复杂的应用程序需要将LLM串联在一起,需要多LLM协同工作。 简介 ------------ tn2>大多数LLM应用都具有对话界面。 对话的一个重要组成部分是对话历史中的信息。 我们将这种存储对话历史中的信息的能力称为`记忆`。 LangChain 提供了一系列记忆相关的实用工具。 这些工具可以单独使用,也可以无缝地集成到一条链中。 >记忆组件需要支持 - 读取 - 写入 tn>注:每条链定义了核心执行逻辑,期望某些输入。 一些输入来自用户,另一些可能来自记忆组件。 在一次与LLM的交互中,链将与记忆组件交互两次: 1.接收到初始用户输入之后,执行核心逻辑之前,链从记忆组件读取历史,并以此增强用户输入。 2.执行核心逻辑之后,在返回回答之前,链把当前交互的输入和输出写入到记忆中,以便更新对话历史。 LangChain的记忆组件类型 ------------ tn2>记忆组件需要解决两大问题: 历史如何存储? 历史如何查询? 本讲通过 LangChain 提供的三种基本记忆组件类型 `ConversationBufferMemory`,`ConversationBufferWindowMemory`,`ConversationSummaryMemory`,介绍它们对于上述问题的解决方案,并分享使用方法。 在此之前请写写好openai的token,并安装相关包。 ```python import os os.environ['OPENAI_API_KEY'] = ''" ``` ```bash !pip install langchain==0.0.235 --quiet ``` ### ConversationBufferMemory tn2>`ConversationBufferMemory` 是 `LangChain` 提供的记忆组件类, 它如实地在列表中记录对话历史消息。 #### 写入一次对话 tn2>通过 `save_context` 函数来保存用户输入和模型输出。 ```python from langchain.memory import ConversationBufferMemory memory = ConversationBufferMemory() memory.save_context({"input": "hi"}, {"output": "whats up"}) ``` tn2>`ConversationBufferMemory` 的 `chat_memory` 成员变量有一个 `messages` 变量。这是一个消息数组。通过如下代码查看消息对象列表 ```python memory.chat_memory.messages ``` tn2>你应该期望看到如下输出:  当我们需要生成对话历史的文本,作为变量嵌入提示词,可以通过调用函数 `load_memory_variables` 获得字典对象,其中的键 `history` 包含了对话历史的字符串值。如下: ```python memory.load_memory_variables({}) ``` tn2>你应该期望看到如下输出:  tn2>`ConversationBufferMemory` 的实现方式简单,在交互次数少,输入输出字符量不大的情况下,非常有效。 但是当交互增加,字符数量增多,对话历史的字符数可能导致增强后的提示词tokens数超过上下文限制,最终导致模型调用失败。 因此,`LangChain` 还提供了其他记忆组件类型。 ### ConversationBufferWindowMemory tn2>`ConversationBufferWindowMemory` 持续记录对话历史,但只使用最近的K个交互。 这种滑动窗口的机制,确保缓存大小不会变得过大。 我们指定滑动窗口的大小为`1`,表示查询时只返回最近`1`次交互。 用法如下: ```python memory = ConversationBufferWindowMemory( k=1) memory.save_context({"input": "Hi, LangChain!"}, {"output": "Hey!"}) memory.save_context({"input": "Where are you?"}, {"output": "By your side"}) ``` tn2>通过 `load_memory_variables` 读取记忆。 ```python memory.load_memory_variables({}) ``` tn2>你应该期望看到如下输出:  tn2>我们看看记忆组件中存储的历史交互: ```python memory.chat_memory.messages ``` tn2>输出:  tn2>可见,组件记忆了所有交互,但是在查询时通过滑动窗口返回指定数量的交互(输入与输出)。 ### ConversationSummaryMemory ```bash # 安装openai包 !pip install openai ``` tn2>`ConversationSummaryMemory` 是稍微复杂的记忆类型。 这种记忆随着时间的推移总结对话的内容,并将当前的摘要存储在记忆中,然后在需要的时候将对话摘要注入提示词或链中。 `ConversationSummaryMemory` 对于更长的对话交互很有用,因为将过去的历史记录逐字逐句放入提示词中会占用太多Token。 tn>注意:由于需要对于对话历史进行总结,生成摘要,因此 `ConversationSummaryMemory` 需要LLM的配合。 我们在示例代码中将提供OpenAI的模型给 `ConversationSummaryMemory` 以生成摘要。 简单来说:它会加以思考的连贯起来,但花的钱(token)也多。 tn2>用法如下: ```python from langchain.memory import ConversationSummaryMemory from langchain.llms import OpenAI memory = ConversationSummaryMemory(llm=OpenAI(temperature=0, openai_api_key="您的有效openai api key")) memory.save_context({"input": "Hi, LangChain!"}, {"output": "Hey!"}) memory.save_context({"input": "How to start with Next.js development?"}, {"output": "You can get started with its official developer guide."}) memory.save_context({"input": "Show me the link of the guide."}, {"output": "I'm looking for you now. Please stand by!"}) memory.load_memory_variables({}) ``` tn2>你应该能看到如下输出:  tn2>你可能注意到了,从记忆组件中得到的对话历史的文本,相较于原始的对话文字,并没有显著地缩短。 原因在于对话的交互只有3次,在这种情况下,摘要的优势并没有显示出来。<br/> 下图是不同记忆类型组件随着对话交互的增加,生成的对话历史信息的Token开销趋势。 可见,`ConversationSummaryMemory` 的Token开销相对平缓,这对于交互多的对话是更有效的。 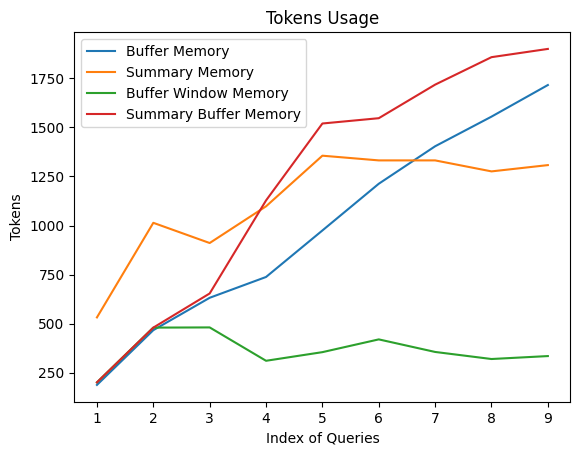 tn2>图中,还展示了我们并没有介绍的类型 `Summary Buffer Memory`。 顾名思义,这是结合了 `Summary` 和 `Buffer` 的优势的一种记忆类型。 ### 与LLM的交互 ```python from langchain.memory import ConversationSummaryMemory, ChatMessageHistory from langchain.llms import OpenAI from langchain.prompts import PromptTemplate from langchain.chains import LLMChain # 定义对话模板 template = """You are a chatbot having a conversation with a human. {conversation_history} Human: {input} Chatbot:""" # 创建对话模板对象 prompt = PromptTemplate( input_variables=["conversation_history", "input"], template=template ) # 创建对话历史内存对象 memory = ConversationBufferMemory(memory_key="conversation_history") # 创建聊天机器人链 llm_chain = LLMChain( llm=OpenAI(), prompt=prompt, verbose=True, memory=memory, ) ``` ```python llm_chain.predict(input="Where is Paris?") ``` ```python llm_chain.predict(input="What did I just ask?") ``` 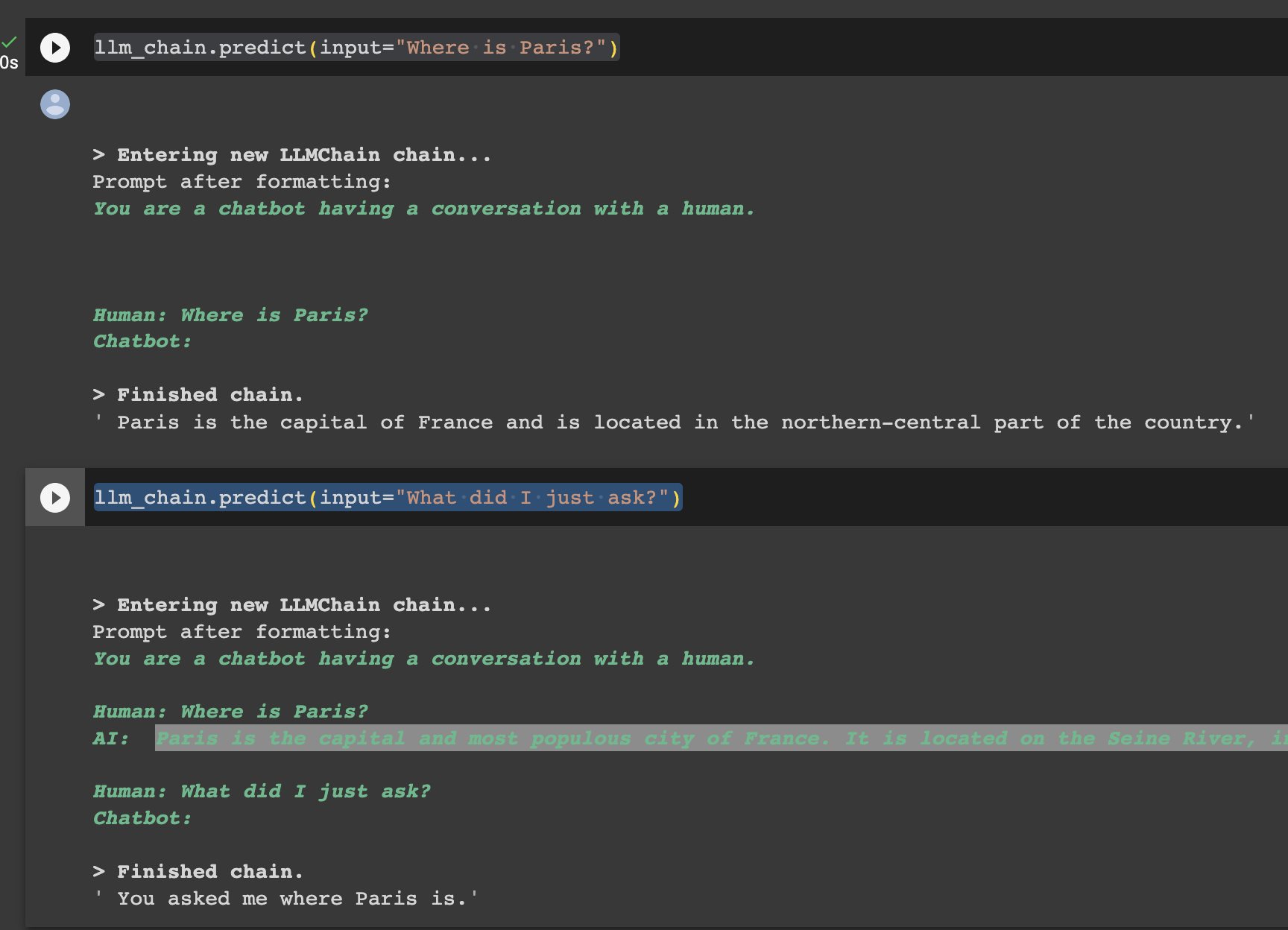 ## 总结 tn2>本节课程中,我们学习了什么是 `记忆组件` ,并通过三种基本记忆组件类型 `ConversationBufferMemory`(不动脑壳的回忆AI回答),`ConversationBufferWindowMemory`(动得不多的回忆AI回答),`ConversationSummaryMemory`(动脑壳的回忆AI回答,但也烧脑壳),介绍它们的工作原理和使用方法。 本课只介绍了 `LangChain` 提供的部分记忆组件,更多类型请参考官方文档 [Memory Types](https://python.langchain.com/docs/modules/memory/types/)。 ### 相关文档资料链接: tn2>1.[Python Langchain官方文档](https://python.langchain.com/docs/get_started/introduction.html) 2.[记忆组件](https://python.langchain.com/docs/modules/memory/) 3.[五里墩茶社](https://space.bilibili.com/615957867?spm_id_from=333.337.0.0)